The annual NHR Conference 2024 is aiming at promoting scientific exchange among the HPC-user community. Each year the focus will be on different scientific topics.
The NHR Conference ’24 took place in Darmstadt. During the Scientific Part users of the NHR Centers had the opportunity to present their projects in a contributed talk or poster session, and to exchange ideas with the consulting and operational teams of the NHR Centers.
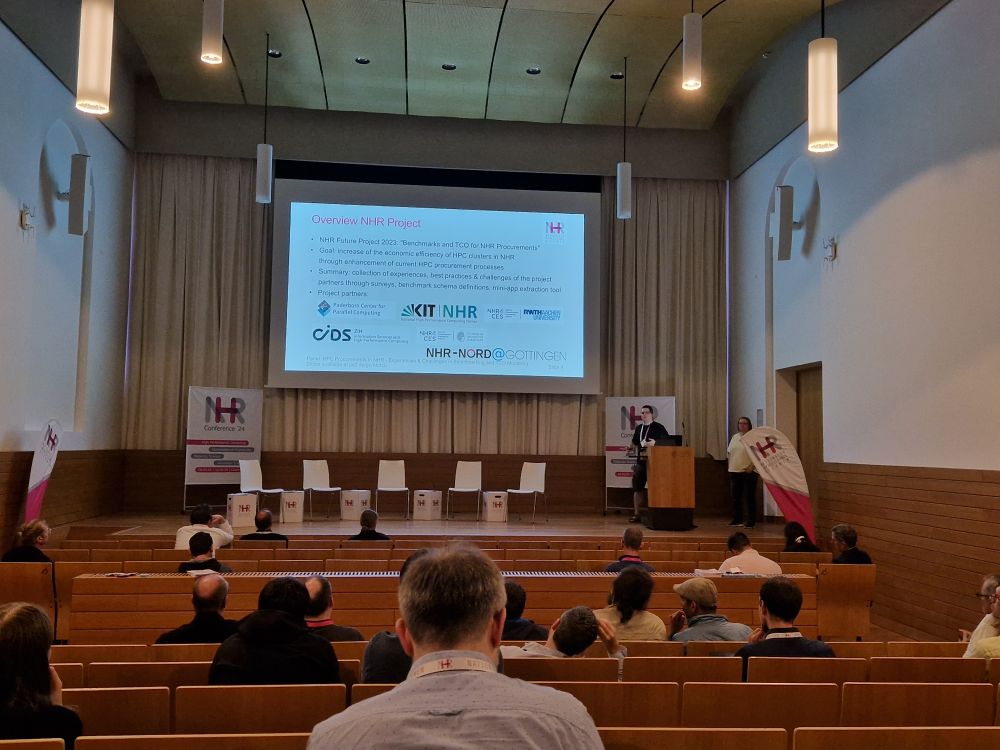
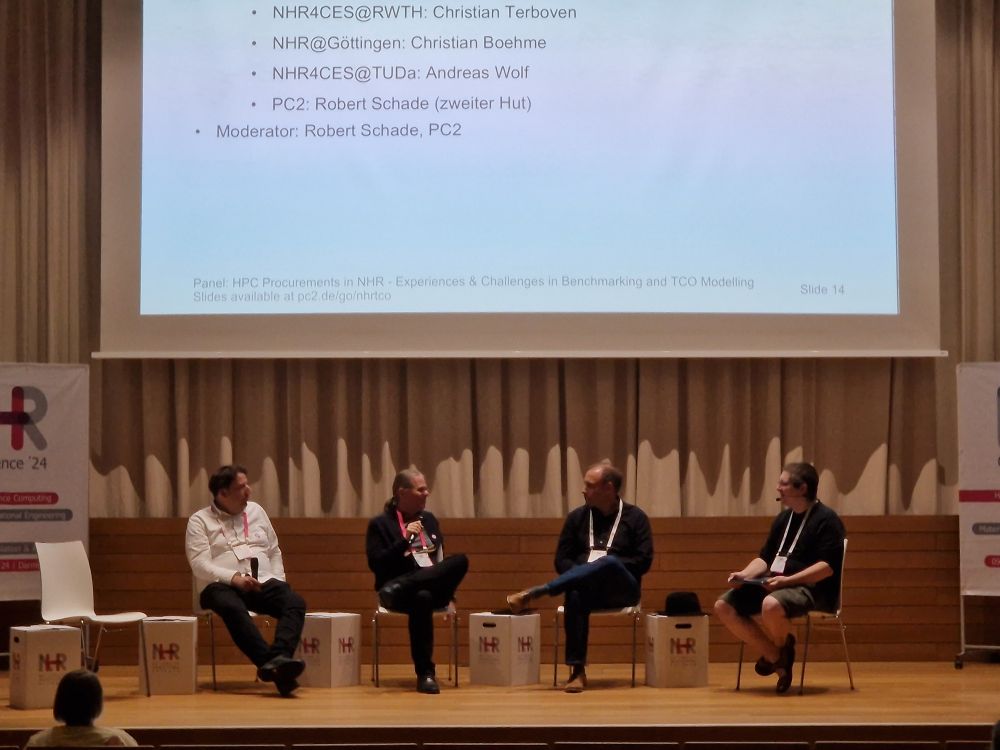
Have a look at our contributions to the NHR Conference 2024:
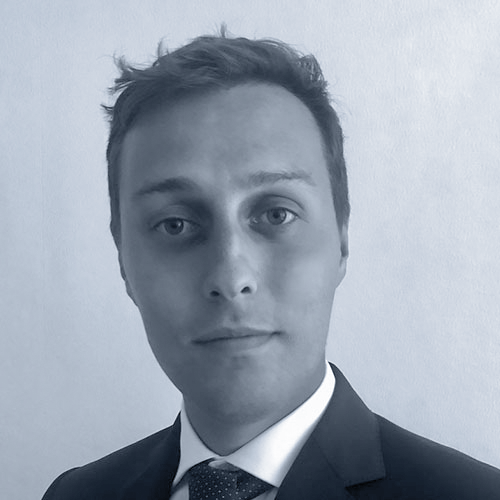
Ludovico Nista
is a research assistant at the Institute for Combustion Technology of the RWTH Aachen University. He has been a research fellow at Von Karman Institute for Fluid Dynamics and visiting scholar at Technion – Israel Institute of Technology, after receiving his Master of Science in Mathematical Engineering and obtaining a Master of Research in Fluid Mechanics at the Von Karman Institute for Fluid Dynamics.
His main research interests are in the fields of machine learning theory, turbulent combustion, and high-order numerical methods with application to applied energy systems.
Since 2020, Ludovico is a member of the SDL Energy Conversion for the National High Performance Computing Center for Computational Engineering Sciences (NHR4CES).
Ludovico Nista’s talk: “Scalability and performance analysis of training and inference-coupled simulations of super-resolution generative adversarial networks for turbulence closure modeling” – September 09, 2024, 02:05 pm to 02:20 pm
Super-resolution (SR) generative adversarial networks (GANs) are promising for turbulence closure modeling in large-eddy simulation (LES) due to their ability to accurately reconstruct high-resolution (HR) data from low-resolution (LR) fields. Current model training and inference strategies are not sufficiently mature for large-scale, distributed calculations due to the computational demands and often unstable training of SR-GANs, which limits the exploration of various model structures, training strategies, and loss-function definitions. Integrating SR-GANs into LES solvers for inference-coupled simulations is also necessary to assess their \emph{a posteriori} accuracy, stability, and cost.
We investigate parallelization strategies for SR-GAN training and inference-coupled LES, focusing on computational performance and reconstruction accuracy. We examine distributed data-parallel training strategies for hybrid CPU–GPU node architectures and the associated influence of low-/high-resolution subbox size, global batch size, and discriminator accuracy. Accurate predictions require training subboxes to encompass at least one integral length scale. Care should be placed on the coupled effect of training batch size, learning rate, number of training subboxes, and discriminator’s learning capabilities.
We introduce a data-parallel SR-GAN training and inference library for heterogeneous architectures that enables on-the-fly data between the LES solver and SR-GAN inference at runtime. We investigate the predictive accuracy and computational performance of this arrangement with particular focus on the overlap (halo) size required for accurate SR reconstruction. Similarly, \emph{a posteriori} parallel scaling is constrained by the SR subdomain size, GPU utilization, and reconstruction accuracy, which limit the computational resources for efficient inference-coupled LES. Based on these findings, we establish guidelines and best practices to optimize resource utilization and parallel acceleration of SR-GAN turbulence model training and inference-coupled LES calculations while maintaining predictive accuracy.
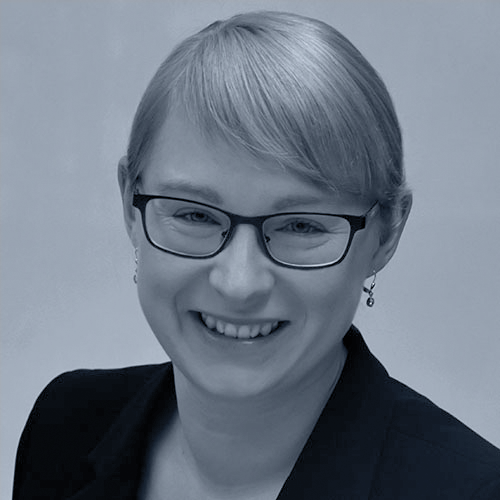
Sandra Wienke
is a research scientist and the deputy manager of the HPC group at the IT Center of RWTH Aachen University. She earned her doctoral degree in computer science from RWTH Aachen University, Germany, for modeling the productivity, total ownership costs and software development efforts in HPC. Her research interests include high-performance computing on parallel heterogeneous architectures. To this end, she is especially interested in the analysis of development productivity, cost effectiveness and performance of benchmarks and real-world applications using various programming models on many- and multicore systems. From day one of NHR (national high-performance computing), she has served as one of the principal investigators of NHR4CES and supports the cross-sectional group “Parallelism and Performance”. Dr. Wienke is part of the Women in HPC (WHPC) community since 2014, and the NHR4CES@RWTH representative of the NHR|WHPC chapter since 2024. Since 2013, she engages in the SPEC High Performance Group (HPG). Furthermore, Dr. Wienke provides support for parallel programming to researchers at RWTH Aachen University and gives lectures and trainings on this topic at universities, workshops and international conferences. She regularly serves on program committees and as organizing committee member for conferences and workshops.
Sandra Wienke presents the NHR strategic project “Benchmarks and TCO for NHR Procurements“- September 10, 2024, 2:30 pm to 4:30 pm
The NHR strategic project “Benchmarks and TCO for NHR Procurements” took place from January 2023 to June 2024 and was a joint work of the NHR centers NHR@Göttingen, NHR@TUD, NHR4CES, PC2 and NHR@KIT. This 10-minute talk presented by project leader Sandra Wienke shows a critical assessment of the project.
This project focuses on benchmarking and TCO modelling for HPC procurements and incorporates the experiences of the six project partners. The project presents experiences, best practices and challenges to integrate individual job mixes in HPC procurements. As part of this approach, the extraction of mini-apps from real HPC applications is eased by using the tool MiniApex.
Furthermore, the partners provide a schema definition for an NHR benchmark collection. In addition, benchmark performance and energy consumption are parameters for informed TCO models used for HPC procurements. The project gives insight to the usage of TCO and productivity models at various HPC sites and, thus, fosters their inclusion to new HPC procurements. Here, the TCO component energy is of particular interest, facing different challenges.
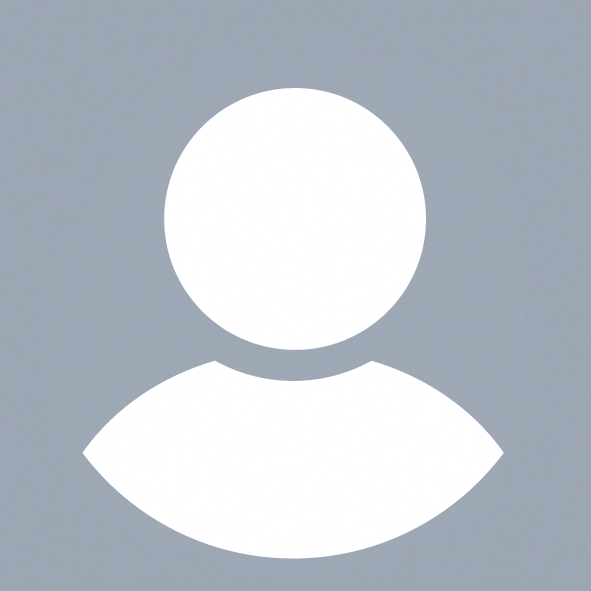
Gustavo de Morais
is a researcher in our CSG Parallelism and Performance.
Gustavo de Morais’ talk: “Performance Modeling for CFD Applications” – September 09, 2024, 10:30 am to 10:45 am – “Computational Engineering Session II”
Understanding performance at scale and identifying potential bottlenecks are crucial for developing and optimizing efficient HPC applications. While computation- and communication-intensive kernels/functions are typically well understood, implicit performance bottlenecks, such as those arising from caching or synchronization effects, can be easily overlooked. Performance models facilitate the identification of scalability bottlenecks. However, designing these models analytically for an entire large code base is often impractical due to the manual effort required. Empirical performance modeling tools, such as Extra-P, allow the automatic creation of performance models for CFD applications and other large software suites, although challenges regarding profiling time and model accuracy arise from their size and characteristics. Based on an exemplary OpenFOAM CFD application, this presentation introduces the concept of strong scaling and provides an overview of common challenges and mitigations of empirical performance modeling. Focusing on large software suites like CFD applications, we demonstrate how to generate and interpret empirical performance models in order to identify potential scalability bottlenecks. For this purpose, we employ the Score-P measurement infrastructure to measure the applications’ performance and Extra-P to generate strong-scaling performance models and identify scalability bottlenecks in the code.
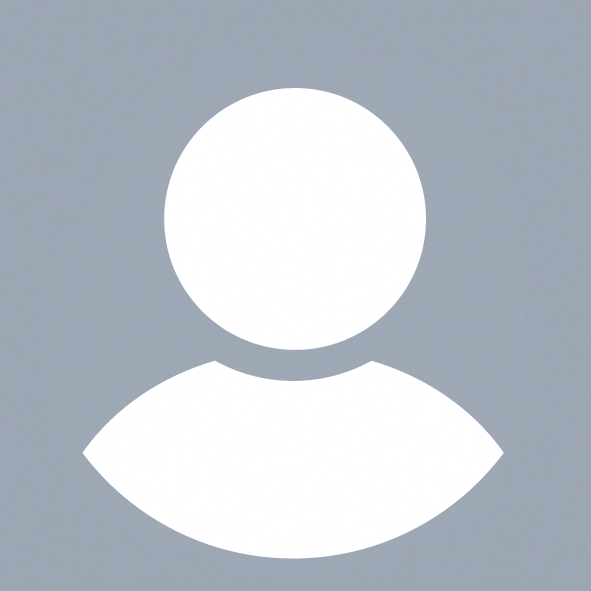
Fabian Orland
received his Bachelor and Master degree in Computer Science from RWTH Aachen University. In August 2019 he joined the chair for high-performance computing at the IT Center of RWTH Aachen Uni-versity as a research assistant and PhD student.
From 2019 until 2022 he was a member of the EU Center of Excellence Performance Optimisation and Productivity (POP2) providing performance assessment services for academic and industrial users from many different scientific disciplines.
Since 2021, Fabian is a member of the Cross-Sectional Group Parallelism and Performance at the Natio-nal High Performance Computing Center for Computational Engineering Sciences (NHR4CES).
Fabian Orland’s talk: “Accelerating Deep Learning Inference in Turbulent Reactive Flow Simulations
on Heterogeneous Architectures” – September 10, 2024, 10:00 am to 10:15 am in the track “Simulation & AI”
Data-driven modeling becomes an increasingly important tool to complement traditional numerical simulations across different domain sciences. In large eddy simulations of turbulent reactive flow, for example, deep learning (DL) models have been successfully applied for turbulence closure, as an alternative to current tabulated chemistry closure and to predict sub-filter scale reaction rates.
In all cases the trained DL model needs to be coupled with a highly parallel simulation code to enable a-posteriori evaluation. This coupling constitutes a computational challenge as heterogeneous architectures need to be exploited efficiently. While traditional numerical simulation codes have been highly optimized to run efficiently on CPUs, the inference of a deep learning model can be significantly accelerated using GPUs or other specialized hardware.
In this talk, we present the AIxeleratorService, an open-source software library developed by us to facilitate the deployment of DL models into existing HPC simulation codes on modern heterogeneous computer architectures. Our library provides users with a modular software architecture abstracting from concrete machine learning framework APIs. Moreover, it integrates seemlessly into the MPI parallelization of a given simulation code and hides necessary data communication between CPUs and GPUs to enable acceleration of the DL model inference in a heterogeneous job.
The AIxeleratorService has been sucessfully applied to above use cases and coupled with popular simulation codes like OpenFOAM and CIAO. We will present a selection of results regarding scalability and speedup on GPUs.
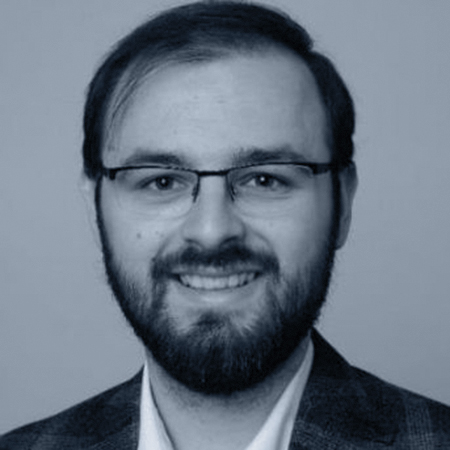
Paul Wilhelm
holds a Bachelor and Master degree in Mathematics with a Minor in Physics obtained at the RWTH Aachen. In his Master thesis he researched the extension of the Bogoliubov scalar product to manifolds of non-quadratic matrices and the existence of gradient flows in the induced geometry. After his Master, Paul worked at keenlogics GmbH in Aachen as a soft-ware engineer developing process optimisation software.
Since 2020, Paul is a PhD student at the Institute of Applied and Computational Mathematics (AcoM) at RWTH Aachen and since 2022 also part of the NHR graduate school. His research focusses on developing new methods for the Vlasov-Poisson equation and, in particular, methods avoiding explicit meshing of the phase-space.
Rotislav-Paul Wilhelm’s talk: “Towards using the Numerical Flow Iteration to simulate kinetic plasma physics in the full six-dimensional phase-space” – September 10, 2024, 9:30 am to 9:45 am – “Computational Engineering Session II”
“High-temperature plasmas can be accurately modelled through the Vlasov equation 𝜕𝑡 𝑓 + 𝑣 · ∇𝑥 𝑓 + 𝑞(𝐸 + 𝑣 × 𝐵) · ∇𝑣 𝑓 = 0, (1) where 𝑓 is a time-dependent probability distribution in the six dimensional phase- space. [1]
The Vlasov equation is non-linearly coupled to the Maxwell’s equations to compute the electro-magnetic forces which are induced through the quasi freely moving charged particles in a plasma. Additionally to the high dimensionality of the problem, the non-linear coupling to the Maxwell’s equations introduces turbulences as well as fine structures called filaments. Thus the challenge is to resolve complicated dynamics with fine but physically relevant structures while working in a high-dimensional setting.
Most schemes to solve the Vlasov equation rely on a direct discretization of the phase-space either using particles or a grid-based approach. This comes with the draw-back of extensive memoryusage making these approaches heavily memory-bound. In particular, in the six-dimensional case only low-resolution simulations can be run with significant overhead in terms of communication, leading to sub-optimal scaling results.[2],[3] The Vlasov equation is strongly transport-dominated thus it is possible to use an iterative-in-time approach to discretize the phase flow and evaluate 𝑓 indirectly. This algorithm, the Numerical Flow Iteration (NuFI), essentially shifts complexity from memory-access to computation-on-the-fly.[4] Only the lower-dimensional electromagnetic potentials have to be stored thus the approach has a low memory footprint even in the full six-dimensional case. Additionally it is embarrassingly parallel, as can be demonstrated using the PoP metrics.popmetrics. However, the low memory footprint comes at the cost of the computational complexity being quadratic in the total number of time-steps instead of linear.
Due to the high degree of structure-preservation through NuFI it is an interesting tool for theoretical investigations of complicated phases in kinetic plasma dynamics. In this work we want to investigate its suitability for more complicated, realistic settings keeping the aforementioned performance-relevant aspects in mind.”
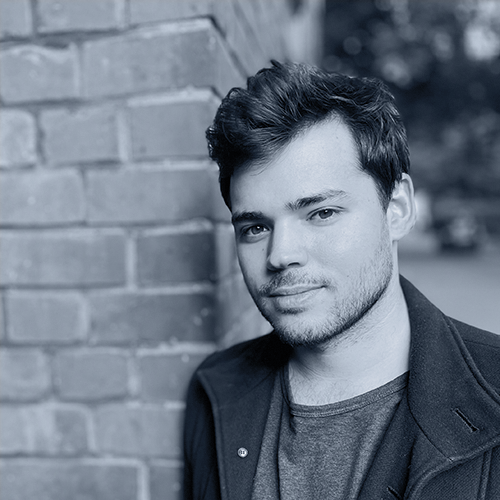
Tim Gerrits
holds a Bachelor and Master degree in Computervisualistics from the University of Magdeburg, Germany, where he also received his PhD in Visualization, working on the visualization of second-order tensor data and vector field ensembles.
From 2019 until 2021, he worked as a postdoctoral researcher at the University of Münster, Germany, with a focus on Visual Analytics approaches for ensemble and uncertain data.
Since 2021, Tim leads the Crosssectional Group Visualization at the National High Performance Computing Center for Computational Engineering Sciences (NHR4CES) as well as the Visualization Group at RWTH Aachen University, Germany.
Tim Gerrits’s talk: “DaVE”
DaVE serves as a centralized repository where users can find and discover visualization examples tailored to their specific needs through a simple search. Our database is designed to be user-friendly, offering seamless integration into existing workflows using adaptable containers. Whether you’re exploring cutting-edge visualizations for data or seeking practical solutions to enhance your simulations, DaVE seeks to find helpful resources for you.
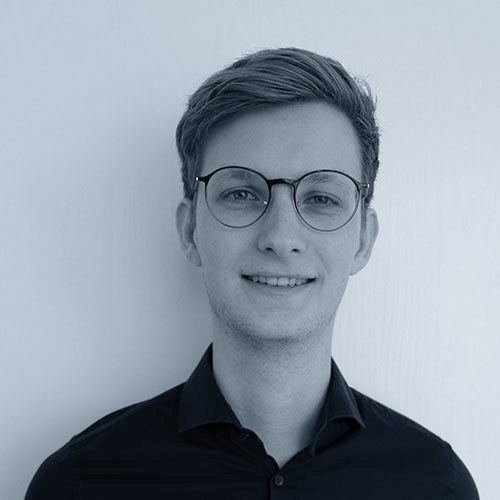
Janis Sälker
is researcher in our SDL Materials Design.
Janis Sälker presented a poster: “Computer vision-based analysis of atom probe tomography data”
Atom probe tomography (APT) is a powerful technique to analyze materials at the nanometer-scale, offering 3D spatially-resolved compositional characterization.
Each measurement can capture up to hundreds of millions of atoms, making data interpretation both time-consuming and operator-dependent. To address these challenges, two deep learning-based analysis methods are being explored. The first one involves supervised image segmentation on 2D representation of APT data to investigate phase changes during thermal decomposition of (V,Al)N thin films. The second method employs an unsupervised contrastive-based strategy to group phase regions with less supervision and input from users.
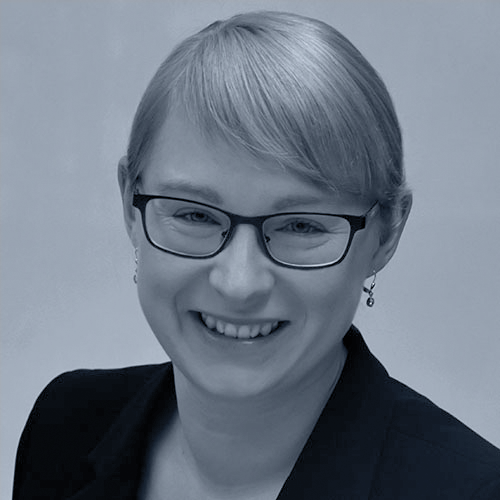
Sandra Wienke
is a research scientist and the deputy manager of the HPC group at the IT Center of RWTH Aachen University. She earned her doctoral degree in computer science from RWTH Aachen University, Germany, for modeling the productivity, total ownership costs and software development efforts in HPC. Her research interests include high-performance computing on parallel heterogeneous architectures. To this end, she is especially interested in the analysis of development productivity, cost effectiveness and performance of benchmarks and real-world applications using various programming models on many- and multicore systems. From day one of NHR (national high-performance computing), she has served as one of the principal investigators of NHR4CES and supports the cross-sectional group “Parallelism and Performance”. Dr. Wienke is part of the Women in HPC (WHPC) community since 2014, and the NHR4CES@RWTH representative of the NHR|WHPC chapter since 2024. Since 2013, she engages in the SPEC High Performance Group (HPG). Furthermore, Dr. Wienke provides support for parallel programming to researchers at RWTH Aachen University and gives lectures and trainings on this topic at universities, workshops and international conferences. She regularly serves on program committees and as organizing committee member for conferences and workshops.
Sandra Wienke – togehter with other projects partners – preseneds the panel “HPC Procurements in NHR – Experiences & Challenges in Benchmarking and TCO Modelling“ – September 12, 11:00 am – 12:00 pm
HPC procurement processes play a major role in making informed decisions on the economic efficiency and suitability of HPC clusters in NHR. The goal of this panel is to foster the exchange and discussion of experiences, best practices, and challenges
on HPC procurements in NHR, and in particular, on benchmarking and Total-Cost-of-Ownership (TCO) modelling as part of requests for proposals (RFPs) and acceptance tests. Furthermore, the panel aims to disseminate this knowledge to further NHR and non-NHR centers, and as such help to ease and enhance current HPC processes.
To this end, the panel targets any staff involved in HPC procurements as participants. The panelists come from the NHR centers – mainly involved in the NHR Strategic Project 2023 “Benchmarks and TCO for NHR Procurements” (whose members also organize this panel). The panel will take place for 60 minutes from which 15 minutes will be used for an overview of the findings of the NHR project “Benchmarks and TCO for NHR Procurements”, 20 minutes for prepared questions, and 25 minutes for questions from the audience and further discussion.
Presenters will be Sandra Wienke (NHR4CES) and Robert Schade (PC2). Robert Schade will also be the moderator of the actual panel. The panelists will be Christian Terboven (NHR4CES), Christian Boehme (NHR@Göttingen), Andreas Wolf (NHR4CES) and Robert Schade (PC2).
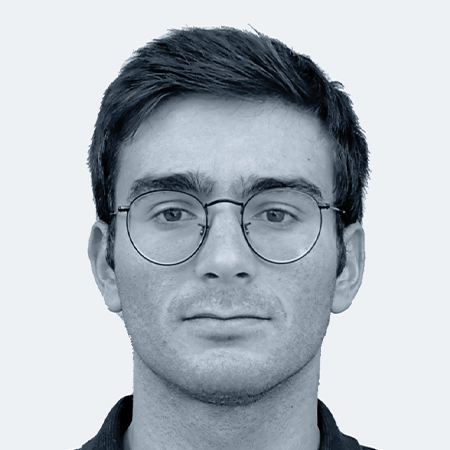
Marco Vivenzo
is researcher in our SDL Energy Conversion.
Marco Vivenzo presented a poster: “Development and Assessment of an External GPU-based Library to Accelerate Chemical Kinetics Evaluation of Reactive CPU-based CFD Solvers”
To facilitate the transition to carbon-free energy conversion systems, high-performance computational fluid dynamics (CFD) codes that leverage heterogeneous current Tier-0 cluster architectures are crucial for combustion system redesigns. While the path to exascale performance lies in GPU utilization, many established reactive CFD codes remain CPU-based only. This is because porting an existing code to a GPU-capable programming language is not a straightforward task, as it might require the redesign of numerical algorithms and extensive recoding. Therefore, a drop-in solution for enabling the usage of new GPU-accelerated systems, without significant changes in the original code, involves the execution of the most time-consuming tasks on GPUs employing easily linkable external libraries.
In this regard, the evaluation of chemical source terms emerges as an optimal candidate for GPU porting.
When operator-splitting schemes are used for the solution of the reactive Navier-Stokes equations, the integration of the stiff ODE system containing the source terms associated with chemical kinetics proves to be the most computationally expensive part. Although the potential of GPUs to accelerate reactive CFD simulations is widely acknowledged, a readily usable library for chemical kinetics capable of harnessing the computational power offered by GPUs is currently absent.
The focus of this work is to develop and assess a C++/CUDA-based library, capable of efficiently integrating chemical terms on GPUs. A comprehensive analysis of the performance and the scaling of the proposed approach over multiple computing nodes will be presented, demonstrating how to accelerate reactive CFD simulations through the integration of external GPU-based libraries.
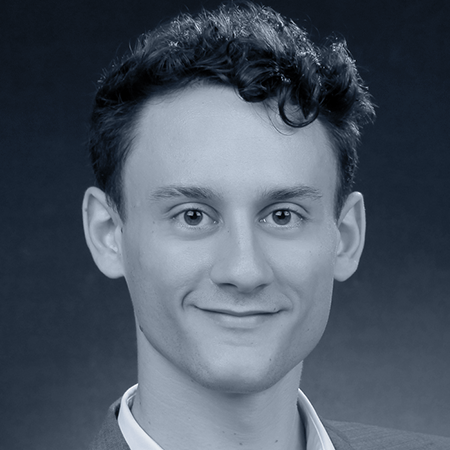
Driss Kaddar
is a research assistant at the department Simulation of reactive Thermo-Fluid Systems of the TU Darmstadt. He received his Master of Science in Chemical Engineering after graduating from the Karlsruhe Institute of Technology.
His main research interests are in the fields of high-performance computing and large-scale simulations of turbulent reactive flows with application to sustainable energy systems.
Since 2021, Driss is a member of the SDL Energy Conversion for the National High Performance Computing Center for Computational Engineering Sciences (NHR4CES).
Driss Kaddar presented a poster: “Ammonia-hydrogen combustion modelling enabled by high-performance GPU computing” (with Hendrik Nicolai, Mathis Bode and Christian Hasse)
Ammonia-hydrogen blends will play a pivotal role for future carbon-free combustion systems. To minimize remaining emissions in ammonia combustion, staged-combustion systems, such as rich-quench-lean technologies, are proposed. However, the combustion behavior of turbulent rich ammonia-hydrogen mixtures lack comprehensive understanding. In particular, the quantification of complex phenomena like partial cracking, hydrogen slip, and post-flame stratification and their interaction with flame structures and pollutant formation remains insufficient.
This is a major scientific barrier hindering the realization of NH3/H2 blends for carbon-free combustion. Recent HPC advancements, particularly in GPU-based systems, enable combustion DNS beyond academic configurations. Utilizing nekCRF, a new GPU-based spectral element solver based on nekRS, we perform finite-rate chemistry DNS of a rich, turbulent premixed jet flame configuration at atmospheric pressure.
This unique data set provides fundamental insights into the intricate interaction of reactions and turbulence that are crucial for developing future models. The analysis focuses on NH3/H2 interaction, revealing residual H2, minimized NH3 slip, and enhanced heat release through turbulent mixing.
We demonstrate the scalability of the spectral element solver on European pre-exascale HPC systems and showcase the implications of a highly scalable GPU-code on the design of sustainable energy solutions.
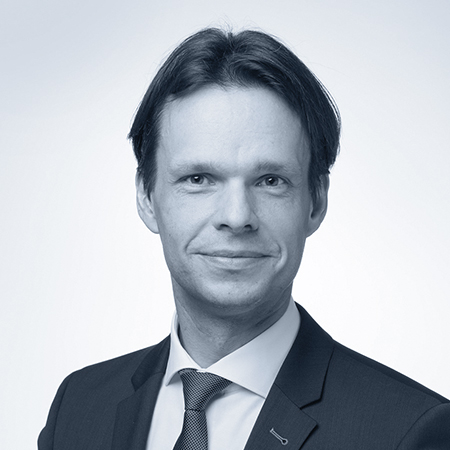
Prof. Dr. Carsten Binnig
is a member of the Department of Computer Science at Technische Universität Darmstadt and Visiting Researcher at Google Systems Research Group.
Prof. Dr. Carsten Binnig’s talk: “Towards LLM-augmented Database Systems” – September 10, 9:00 am – 9:30 am
Recent LLMs such as GPT-4-turbo can answer user queries over multi-model data including tables and thus seem to be able to even replace the role of databases in decision-making in the future. However, LLMs have severe limitations since query answering with LLMs not onlyhas problems such as hallucinations but also causes high-performance overheads even for small data sets. In this talk, I suggest a different direction where we use database technology as a starting point and extend it with LLMs where needed for answering user queries over multi-model data. This not only allows us to tackle problems such as the performance overheads of pure LLM-based approaches for multi-modal question-answering but also opens up other opportunities for database systems.
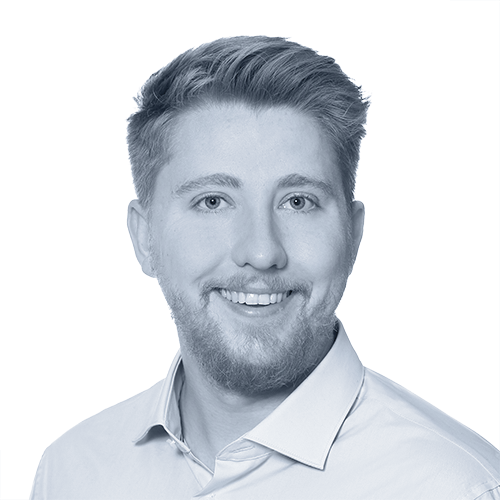
Tobias Bongartz
holds a Bachelor’s degree in Computational Engineering Science from RWTH Aachen University, during which he undertook his externship at the ABB Corporate Research Center in Baden, Switzerland. Following this, he completed his Master’s degree in Computational Engineering Sciences at RWTH Aachen University, where he researched discontinuous Galerkin methods and non-equilibrium gas dynamics as his area of interest.
Since 2021, Tobias has been working as a Scientific Coworker at the Chair of Computational Analysis of Technical Systems (CATS) at RWTH Aachen University and as a member of the Simulation and Data Lab for Fluids of the SDL Fluids National High-Performance Center for Computational Engineering Sciences (NHR4CES). His research includes stabilized finite element methods to model pathological blood clotting for the development of next-generation anticoagulants in biomedical sciences.
Tobias Bongartz’ talk: “Space-Time Finite Element Methods for Investigating the Role of Prothrombin Mutations in Thrombus Formation” – September 09, 1:50 pm – 3:05 pm (with Alessia Piergentili, Giulia Rossetti, Marek Behr)
The inherent hemostatic response to vascular injury prevents blood loss, but excessive thrombosis may impede blood flow to vital organs or tissues [1]. As an essential stage of the coagulation mechanism, prothrombin is activated to thrombin. Thrombin is then involved in the activation of blood platelets, the production of fibrin, and an amplification mechanism of the coagulation. As recently shown in [2], in equilibrium prothrombin exists between two forms: “closed” (~80%) and “open” (~20%). The binding of prothrombin to prothrombinase occurs primarily in the closed form, allowing for a slightly more efficient conversion to thrombin. Thus, the ratio between the two forms of prothrombin is a key determinant for blood clotting and an imbalanced ratio may be associated with pathologies. In this work, we present a mathematical model for the prediction of localized thrombus formation which covers the mechanisms of the human blood coagulation process. The model will take into account the impact of the changes in prothrombin open/close ratio on blood clotting upon prothrombin mutations and ligand binding. This aspect is not taken into account by mathematical models currently available in the literature, e.g., as shown in [3]. Within the model, a set of convection-diffusion-reaction (CDR) equations is coupled to the incompressible Navier-Stokes equations. Endothelial injuries/dysfunctions are modeled with boundary conditions to the above equations. We solve our model using a stabilized space-time finite element method [4] and apply this to test cases with realistic blood-flow conditions and vessel geometries. Importantly, we will discuss the role of High Performance Computing within the framework of NHR4CES and highlight the performance and accuracy enhancements for this method.
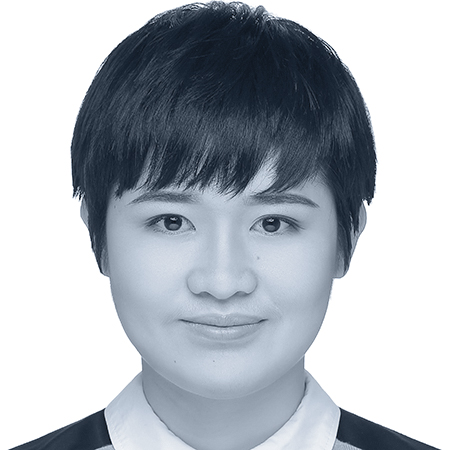
Xiaoyu Wang
is a researcher at TU Darmstadt, Institute for Fluid Mechanics and Aerodynamics.
Xiaoyu Wang’s talk: “Resolution assessment in sensitized RANS modeling of concentric annular flows” – September 09, 1:50 pm – 3:05 pm (with Suad Jakirlic)
A concentric annular turbulent flow, both with and without inner wall rotation is computationally simulated using a conventional differential, near-wall Reynolds Stress Model (RSM) [1] and its eddy-resolving version, sensitized appropriately to account for the turbulence unsteadiness (referred to as the Improved Instability-Sensitized RSM model –IIS-RSM [2]) within the unsteady Reynolds-Averaged Navier-Stokes (RANS) computational framework. The computational study is performed over a range of rotation rates (N = 0, 0.2145, 0.429, 0.858 and 1.716) at Reynolds number ReDh = 8900 and radius ratios (curvature parameters) = Ri⁄Ro = 0.01, 0.1 and 0.5. The length scale-governing equation describing the inverse turbulent time scale relies on the so-called ‘homogeneous dissipation’ rate. The eddy-resolving capability of the IIS-RSM is achieved by selectively enhancing the turbulence production by introducing an additional production term in the scale-determining transport equation, in accordance with the Scale-Adaptive Simulation (SAS) methodology [3]. The primary objective of the present contribution is to evaluate how the computational domain size, rotation intensity, and the radius ratio affect the resolution ratio, specifically the ratio of modeled to total Reynolds stress components according to the equations of the IIS-RSM. This evaluation is undertaken based on the high-level agreement with the available reference data from Direct Numerical Simulation (DNS) and Large Eddy Simulation (LES) studies [4][5].
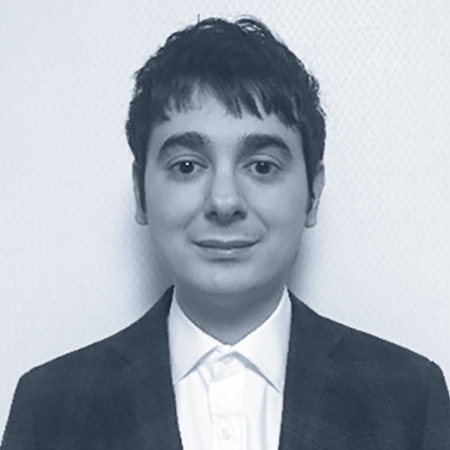
Vasilios Karanikolas
holds a BSc and MSc from University of Patras in Greece studying theoretical high energy physics. In 2016 he received his PhD from Trinity College Dublin in Ireland, where he focused on designing light emitting and harvesting devices using numerical and semi-analytical meth-ods.
From 2019 to 2022 he was a ICYS Fellow at NIMS Japan. He developed an ultra-fast and ultra-bright quantum photon source, applying state-of-the-art numerical, theoretical and experi-mental techniques.
Since the April of 2023 he joined the Materials Institute of TU Darmstadt to apply machine learning protocols to perform large scale atomistic simulations.
Vasilios Karanikolas’ talk: “Investigating Thin-Film Solar-Cell Absorbers with a Machine Learning Potential” – September 09, 1:50 pm – 3:05 pm (with Delwin Perera, Karsten Albe)
Nowadays, applying machine learning (ML) techniques in high performance computing infrastructures allow us to tackle large-scale complicated problems in many fields of science
and engineering. We apply such techniques to design the next generation solar cell devices. While the majority of solar cell technology is still dominated by silicon-based cells, these cells are reaching an efficiency plateau of around 25%. This plateau may be overcome by Cu-InGa-Se (CIGS) thin film solar cells. Due to thicknesses of a few hundred nanometers these thinfilms can be used on flexible substrates creating new applications. Currently, their efficiency is around 21%. Various routes have been devised to enhance this value for instance by replacing Cu with Ag. The exact physical mechanisms involved are, however, elusive. In this work, we investigate the thermodynamic properties of Ag(1−x)Cu(x)GaSe2 structures based on an ML interatomic potential. The training dataset for the regression ML model is created by density functional theory calculations. The ML potential provides the total energy of the structure under investigation and the potential landscape felt by each atom within the structure. We use the ML potential to perform molecular dynamics simulations for large and complex structural environments, inaccessible to conventional electronic structure methods. Moreover, we analyze the diffusion of Cu and Ag between a CuGaSe2/AgGaSe2 interface, where a vacancy defect is included in each side of the interface. Results are compared to experimental values of the diffusion coefficients. In summary, ML potentials are computationally efficient and can promote our understanding of complex systems significantly.
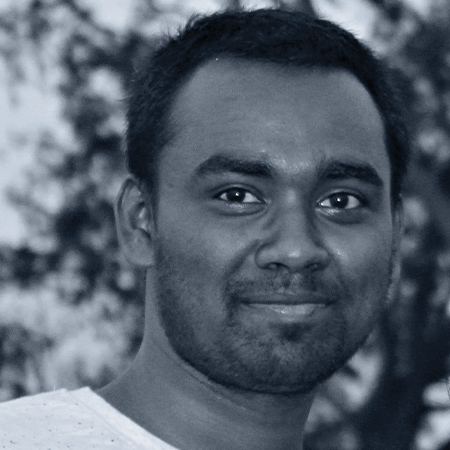
Ganesh Kumar Nayak
is trained as a physicist with a Bachelor’s and Master’s degree in Physics from the Fakirmohan University, India and Central University of Punjab, India respectively. He was awarded a PhD in computational material science from Montanuniversität Leoben, Austria, working with simulation methods such as ab initio, molecular dynamics, and the fitting of interatomic potential by machine learning to simulate disorder materials.
Since January 2023, Ganesh has been a postdoctoral researcher at Department of Materials chemistry RWTH Aachen University, Germany. He continues his research on simulation of materials by fitting of machine learning interatomic potential and investigating the structure-property relationships in materials by machine-learning.
Ganesh Kumar Nayak’s talk: “Insights into thermal stability and decomposition of Ti1−xAlxB2 by combining ab initio and machine learning-assisted molecular dynamics” – September 10, 9:30 am – 11:00 am (with Jochen M Schneider)
Titanium diboride (TiB2) is a compound known for its high melting point, thermal stability, and hardness. However, due to oxidation, its application in the atmosphere is limited to 400 ⁰C. The addition of Al into TiB2 has been found to enhance oxidation resistance, which could significantly expand the practical applications of this material. This is because it triggers the formation of Al-containing oxide scales with early diffusion of Al compared to Ti. However, the Al diffusion can lead to segregation at grain boundaries and hence the phase separation, a process that we aim to understand better in the context of the thermal stability of Ti1−xAlxB2. Ab initio calculations limit the simulation of diffusion and, hence, the phase separation during annealing due to the restriction of the system size and time scale. Large-scale simulations such as molecular dynamics (MD) can be employed to tackle these restrictions. However, the forcefield to describe the interactions is a challenge in such simulations. In the context of forcefield, machine learning (ML)-based interatomic potentials offer a unique advantage in enabling simulations of extended systems. Their accuracy is largely comparable to DFT, but with a computational cost that is significantly lower by several orders of magnitude. Furthermore, MD simulations demonstrate favorable linear (order N) scaling behavior, enhancing the efficiency
of simulations. In this contribution, we employ an approach that includes ab initio and ML potential-assisted MD simulations to study the Al concentration dependent thermal stability of Ti1−xAlxB2 Our approach to quantum-mechanical calculations, combined with the special quasi-random structure (SQS) method, allows us to position all atoms precisely. This unique statistical approach enables us to measure activation energies for vacancy-driven migration mechanisms, a crucial aspect of the decomposition process. Later, with these ab initio data sets we train an ML-based potential to simulate and understand the onset of decomposition, and phase separation by focusing on the stochiometric Ti1−xAlxB2.
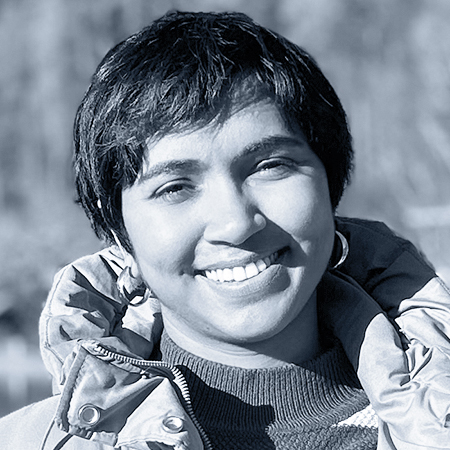
Aditi Mohadarkar
holds a Bachelors Degree of Science (Bsc Mathematics Major) from University of Pune, India.
Masters Degree of Science (MSc Mathematics Major) from Christ university, Bangalore India.
Masters of Mathematics and Computer science (Applied Mathematics) University of Göttingen, Germany.
From 2020-2022 worked as a Student Assistant with Numerics and Applied Mathematics Insti-tute of University of Göttingen. From 2021-2022 worked with MBExC, Stochastic Institute in collabration with University of Medicine Göttingen, with the focus on Mathematical modelling specialising Stochastic processes and Numerical Methods.
In the year 2023 worked as a Intern with Market Data Unit of Deutsche Börse Group Frankfurt.
Since 2023 started working with Material science Institute focus on computational Material Science in desiging of Materials with Machine Learning techniques.
Aditi Mohadarkar’s talk: “Segmentation of Particles in Scanning Electron Microscopy Images: A Comparison of Manual and Automated Methods” – September 09, 1:50 pm – 3:05 pm (with Mozhdeh Fathidoost, Sebastian Wissel, Bai-Xiang Xu)
This study focuses on the segmentation of superposed Lithium nickel manganese cobalt oxides (NMC) particles in scanning electron microscopy (SEM) images, aiming to examine their physical characteristics for optimizing battery performance. The size and shape of particles are known to influence electrode density, porosity, and electrochemical reactivity, thereby impacting battery efficiency and capacity. We compare non-automated and automated algorithmic approaches, utilizing advanced high-performance computing (HPC) techniques. In non-automated methods, we employ the Canny and Sobel edge algorithms for spatial imagery analysis and investigate the efficacy of the watershed object detection algorithm. In SEM, the use of secondary electrons for signal generation leads to complex contrast formation strongly influenced by surfaces, resulting in challenges for non-automated segmentation approaches [?]. Our findings underscore the necessity of automated methods to address these challenges and facilitate the study of particle characteristics, with HPC playing a pivotal role in accelerating computational tasks and improving segmentation accuracy. In the future, we advocate for the widespread adoption of automated approaches, such as Deep learning-based image segmentation algorithms, powered by HPC infrastructure, to enhance the accuracy and efficiency of particle segmentation in SEM images. The proposed workflow involves generating synthetic images that closely resemble the experimental images in both geometric and visual characteristics. These synthetic images are then utilized to train the neural network, with HPC facilitating rapid processing for the training process.
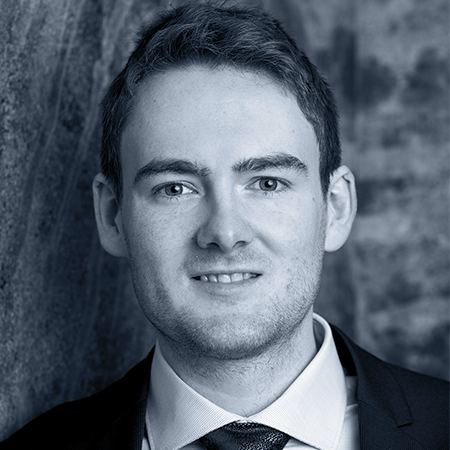
Julian Thull
holds a Bachelor and Master degree in Computer Science from RWTH Aachen Univer-sity. Since 2022 he works at the RWTH Institute of Imaging and Computer Vision, pursuing his PhD in the field of biomedical image processing under supervision of Prof. Dorit Merhof.
Julian Thull presented a poster: “Refining Position Estimates of PET Detector Blocks with Stochastic Gradient Descent” – September 09 & 10 (with Florian Mueller, Volkmar Schulz)
Mechanical misalignments of PET detectors, resulting from manufacturing imprecisions, significantly compromise image quality. Precise position estimation becomes crucial amidst the complexities of evolving whole-body imaging systems and the introduction of new scanner technologies. Traditional methods utilize single point source measurements. We propose an alignment strategy that works for arbitrary tracer distributions. Our method optimizes transaxial alignment parameters to match a differentiable approximation of the sinogram with the analytical solution for tracer line integral distributions, given by the Radon transformation. We utilize the Adam optimizer and use an adaptive normalization strategy and convex hull regularization to counteract effects of missing data due to gaps between detector elements. Our study utilized GATE simulations of a small animal PET scanner with intentionally introduced detector misalignments. We assessed our method’s efficacy by comparing found detector configurations against the ground truth. Results indicate the method’s potential in estimating detector alignment with approximately 300µm precision, albeit with limitations towards y-axis rotations due to their minimal impact on the sinogram. In conclusion, our approach presents a viable solution for PET detector alignment, offering a foundation for future enhancements, including z-axis alignment and additional corrections for effects like attenuation, scatter or randoms.
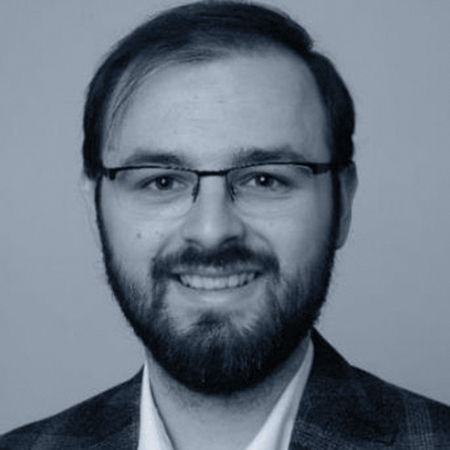
Paul Wilhelm
holds a Bachelor and Master degree in Mathematics with a Minor in Physics obtained at the RWTH Aachen. In his Master thesis he researched the extension of the Bogoliubov scalar product to manifolds of non-quadratic matrices and the existence of gradient flows in the induced geometry. After his Master, Paul worked at keenlogics GmbH in Aachen as a soft-ware engineer developing process optimisation software.
Since 2020, Paul is a PhD student at the Institute of Applied and Computational Mathematics (AcoM) at RWTH Aachen and since 2022 also part of the NHR graduate school. His research focusses on developing new methods for the Vlasov-Poisson equation and, in particular, methods avoiding explicit meshing of the phase-space.
Paul Wilhelm presented a poster: “Discussion of potentials and draw-backs of using the Numerical Flow Iteration to solve
the Vlasov equation” – September 09 & 10 (with Fabian Orland, Manuel Torrilhon)
In the context of high-temperature plasmas the velocity-distribution is often too far away from equilibrium to use fluid-dynamical models, thus one has to resort to the Vlasov equation arising from kinetic theory. However, the Vlasov equation is a non-linear, up six-dimension partial differential equation, which makes classical numerical approaches prohibitively expensive. We present a novel approach, the Numerical Flow Iteration, based on the Lagrangian structure of the Vlasov equation using an iterative scheme to evaluate the characteristic map and thereby the solution on-the-fly. In this work we compare this approach to other numerical solvers for the Vlasov equation in terms of both accuracy and computational efficiency.